Chapter 5 Complete Block Designs ANOVA and Mixed Models
Table Of Content

We can group experimental units into blocks so that each block contains relatively homogeneous units. The design is balanced having the effect that our usual estimators andsums of squares are “working.” In R, we would use the model formulay ~ Block1 + Block2 + Treat. We cannot fit a more complex model, includinginteraction effects, here because we do not have the corresponding replicates.
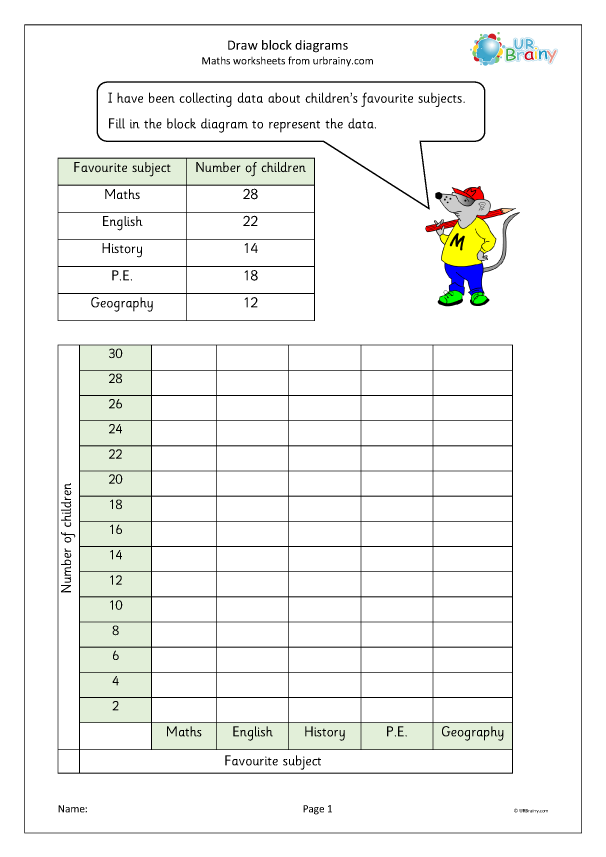
Symmetric 2-designs (SBIBDs)
Without the blocking variable, ANOVA has two parts of variance, SS intervention and SS error. All variance that can't be explained by the independent variable is considered error. By adding the blocking variable, we partition out some of the error variance and attribute it to the blocking variable. As a results, there will be three parts of the variance in randomized block ANOVA, SS intervention, SS block, and SS error, and together they make up SS total. In doing so, the error variance will be reduced since part of the error variance is now explained by the blocking variable.
Designer Related Hirings
Blocking can also be understood as replicating an experimenton multiple sets, e.g., different locations, of homogeneous experimental units,e.g., plots of land at an individual location. The experimental units shouldbe as similar as possible within the same block, but can be very differentbetween different blocks. This design allows us to fully remove thebetween-block variability, e.g., variability between different locations, fromthe response because it can be explained by the block factor. In that sense, blocking is a so-calledvariance reduction technique.
Lesson 4: Blocking
Graphic design has been a staple of digital marketing, website design, and even printable materials since computers were capable of it. Statistics.com prepares the leaders of tomorrow with cutting-edge data science skills that are perfectly suited to the challenges they want to conquer. Statistics.com is powered by Elder Research, a data science consultancy with 25 years of experience in data analytics, and is certified to operate by the State Council of Higher Education for Virginia (SCHEV).
We could put individuals into one of two blocks (male or female). And within each of the two blocks, we can randomly assign the patients to either the diet pill (treatment) or placebo pill (control). By blocking on sex, this source of variability is controlled, therefore, leading to greater interpretation of how the diet pills affect weight loss. For example, an agricultural experiment is aimed at finding the effect of 3 fertilizers (A,B,C) for 5 types of soil (1…5).
Designer gender ratio
Optimising the classification of feature-based attention in frequency-tagged electroencephalography data Scientific Data - Nature.com
Optimising the classification of feature-based attention in frequency-tagged electroencephalography data Scientific Data.
Posted: Mon, 13 Jun 2022 07:00:00 GMT [source]
With a randomized block experiment, the main hypothesis test of interest is the test of the treatment effect(s). A non-blocked way to run this experiment would be to run each of the twelve experimental wafers, in random order, one per furnace run. That would increase the experimental error of each resistivity measurement by the run-to-run furnace variability and make it more difficult to study the effects of the different dosages. The blocked way to run this experiment, assuming you can convince manufacturing to let you put four experimental wafers in a furnace run, would be to put four wafers with different dosages in each of three furnace runs. The only randomization would be choosing which of the three wafers with dosage 1 would go into furnace run 1, and similarly for the wafers with dosages 2, 3 and 4. In complete block design, every treatment is allocated to every block.
An Ultimate Guide to Matching and Propensity Score Matching - Towards Data Science
An Ultimate Guide to Matching and Propensity Score Matching.
Posted: Fri, 18 Jun 2021 07:00:00 GMT [source]
Driving experience in this case can be used as a blocking variable. We will then divide up the participants into multiple groups or blocks, so that those in each block share similar driving experiences. For example, let's say we decide to place them into three blocks based on driving experience - seasoned; intermediate; inexperienced. Often blocking variables are not the variables that we are primarily interested in, but must nevertheless be considered.
Model
A randomized block design with the following layout was used to compare 4 varieties of rice in 5 blocks. One common way to control for the effect of nuisance variables is through blocking, which involves splitting up individuals in an experiment based on the value of some nuisance variable. Here, the condition that any x in X is contained in r blocks is redundant, as shown below. The yield of oats from a split-plot field trial using three varieties and four levels of manurial treatment. The experiment was laid out in 6 blocks of 3 main plots, each split into 4 sub-plots.
Error
Suppose engineers at a semiconductor manufacturing facility want to test whether different wafer implant material dosages have a significant effect on resistivity measurements after a diffusion process taking place in a furnace. They have four different dosages they want to try and enough experimental wafers from the same lot to run three wafers at each of the dosages.

At a high level, blocking is used when you are designing a randomized experiment to determine how one or more treatments affect a given outcome. More specifically, blocking is used when you have one or more key variables that you need to ensure are similarly distributed within your different treatment groups. In the previous example, gender was a known nuisance variable that researchers knew affected weight loss. Gender is a common nuisance variable to use as a blocking factor in experiments since males and females tend to respond differently to a wide variety of treatments. A special case is the so-calledLatin Square design where we have two blockfactors and one treatment factor having \(g\) levels each (yes, all of them!).Hence, this is a very restrictive assumption. In a Latin Square design, eachtreatment (Latin letters) appears exactly once in each row and once ineach column.
But more often than not, is worth it in terms of the improvement in the calculated \(F\)-statistic. In our example, we observe that the \(F\)-statistic for the treatment has increased considerably for RCBD in comparison to CRD. It is reasonable to assume that the result from the RCBD is more valid than that from the CRD as the MSE value obtained after accounting for the block to block variability is a more accurate representation of the random error variance.
The incidence matrix of a non-binary design lists the number of times each element is repeated in each block. First, the blocking variable should have an effect on the dependent variable. Just like in the example above, driving experience has an impact on driving ability. This is why we picked this particular variable as the blocking variable in the first place. Even though we are not interested in the blocking variable, we know based on the theoretical and/or empirical evidence that the blocking variable has an impact on the dependent variable.
Comments
Post a Comment